Interview: SURF pushes the boundaries of deep learning
Deep learning is developing rapidly, partly thanks to the work of SURF. By using alternative technology and smart techniques, we regularly achieve spectacular results, says SURF consultant and team leader High Performance Machine Learning Valeriu Codreanu.
Recognizing tumors with CPUs
Sometimes it helps to do things differently from others. "Everyone uses graphical processors (GPUs) for deep learning, but we don't," says Codreanu. "In close cooperation with Intel, we run the models on CPUs, the central processors of any PC." SURF is an Intel Parallel Computing Center; in this program, universities, research institutes and labs work together with Intel to optimize open-source applications for computing power.
This leads to spectacular results. With an American file of 112,000 radiographs, Codreanu and his colleagues trained a model to recognize tumors in just 8 minutes, with the same average reliability as the best existing models. But that was just the beginning. "We realised that other researchers worked with compressed X-rays because of the limited memory space of GPUs: not 1,000 x 1,000 but 256 x 256 pixels. We didn't have that limitation. When we adapted a model and released it on the full-size photos, reliability soared."
Recognizing 300,000 plants
But there are bigger challenges. For X-rays, the model only had to distinguish 14 types of tumors, but SURF researchers also work with Pl@ntNet. Millions of people worldwide use this app to recognise plants. At the moment, the software distinguishes around 15,000 species of flora, but there are no less than 300,000 plant species in the world.
"In addition," Codreanu explains, "the Plantnet database contains almost twelve million pictures of plants, about one and a half terabytes of data. Too much for the existing computer systems." With two techniques from high performance computing, Codreanu and his colleagues managed to make the material manageable. On a heavy supercomputer in France (which at the time had the latest hardware), they succeeded in increasing the reliability of plant recognition by a third within 16 hours.
The significance of this project goes beyond biology. Codreanu: "You can use these techniques for every domain in which you need to distinguish large numbers of categories. Just think of facial recognition."
Simulation in record time
A completely different application of deep learning was developed by SURF researchers for CERN, the European Organization for Nuclear Research. "They had a problem," says Codreanu. "Simulations are essential for their research with the particle accelerator, but they consume half of their computer capacity. And you should realise that their computing grid is twenty times more powerful than our national supercomputer."
CERN sought the solution in a deep learning application: 'generative adversarial networks'. These are models that can generate new content based on a training set. Codreanu: "They are also used in the media to realistically edit the faces of celebrities: deep fakes. But here the input consisted of data from existing simulations to develop new, deep-learning simulations."
SURF's expertise consisted of scaling up the model without any loss of output. "As a result, we reduced the training time from weeks to hours. In this way, deep learning simulations can be developed and perfected much more quickly."
Combining CT scans
SURF also uses generative adversarial networks in research for the Netherlands Cancer Institute NKI. In radiotherapy, a CT scan is made before treatment. But a treatment takes weeks. During this time, the physical condition of the patient can change and the CT scan is no longer always representative.
During the treatment, however, CT scans are also made daily to verify whether the patient is lying in the right position on the examination table. Unfortunately, these are of insufficient quality to base a new treatment plan on. In the collaborative project, NKI and SURF try to generate CT's of sufficient quality on the basis of the original CT scan and these daily images.
"Our first goal is to achieve the required accuracy in the images," says Codreanu. "This is not easy, because the scans are in 3D: about one gigabyte per scan. Again, the GPUs that are normally used for deep learning are of no use here."
Enormous societal need
These latter applications of deep learning, simulations and medical research, will be central to SURF's work in this area in the near future. Codreanu: "Researchers want to run more and more detailed simulations on our systems. Then you really need new approaches such as deep learning. And, of course, medical research meets an enormous societal need."
To help researchers with the use of deep learning, SURF has set up a High Performance Machine Learning Group of five experts led by Codreanu. "In the end, we have only one goal," he emphasises: "to help Dutch scientists conduct world-class research. And for that, we ourselves must continue to lead the way."
Text: Aad van de Wijngaart
SURF Open Innovation Lab
The deep learning innovations take place in the context of the SURF Open Innovation Lab. Innovation is crucial for SURF and its members to meet major challenges in research, education and society. The SURF Open Innovation Lab brings together all activities and experiments in the field of early innovation and open collaboration. SURF does this in partnership with institutions and the business community.
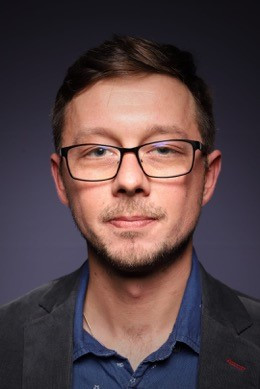
Valeriu Codreanu (1984) was trained as a chip designer in Romania. After his PhD in 2011, he relocated to Groningen. He spent two years as a postdoc at the university exploring the software side of IT with the aim of accelerating GPUs. After this, he worked for a year at Eindhoven University of Technology on embedded computing, focusing on minimum capacity, before - ironically enough - making the switch to the biggest and baddest computer in the Netherlands: SURF's national supercomputer Cartesius.
Over the past five years, he has specialised in deep learning there. "Deep learning may be a black box, but it is a black box that works incredibly well in many cases. That can be invaluable."
Publications and presentations
PlantNet:
- Presentation Towards the recognition of the world’s flora for TERATEC Forum 2018
- Presentation Pl@ntNet: towards the recognition of the world’s flora for Orap Forum 2018
- Presentation Pl@ntNet: towards the recognition of the world’s flora for Digital Infrastructures for Research 2018