Home
SURF is the IT cooperative of education and research
This is how we innovate IT in education and research
-
What you need to know about... certificates
13 minutes
Praat mee op SURF Communities!
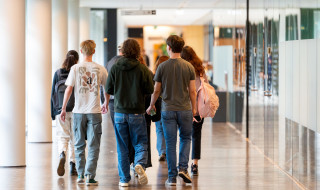
-
Community
Praktijkgericht Onderzoek
66 followersEr wordt steeds meer samengewerkt in het praktijkgericht onderzoek (PO) en dat vraagt om uitwisseling van kennis en best practices! Of het nou gaat over hoe je het beste met onderzoeksdata om kan gaan...
Survive or innovate? The library in a digital world
What does the future of the library look like? What opportunities and threats are there? We discuss with Hilde van Wijngaarden, president of the UKB consortium, and Tecla ten Berge, president of SHB.
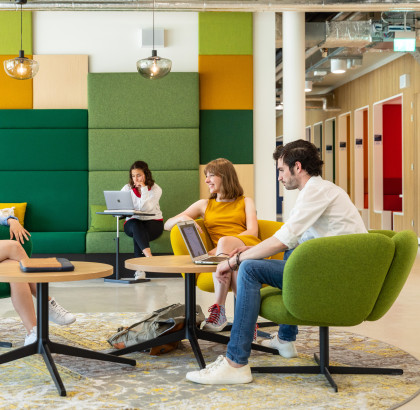
What you need to know about... The AI Factory
Ronald Stolk, founding director of the upcoming AI factory, talks about the importance of autonomy and what this AI factory could mean for us. Listen to the new episode now!
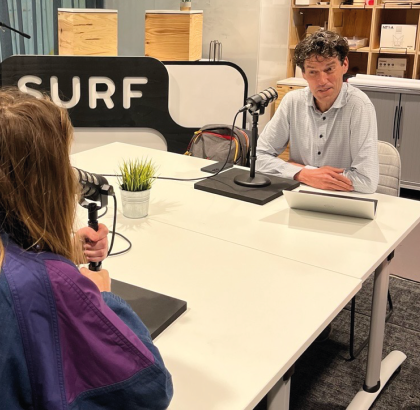
A fight for recognition
How do you embed open science in hbo? Sarah Coombs does so with a mix of expertise, networking, games and tenacity. "I would like to see the DCC-PO fully recognised as a centre of expertise for research support. That we don't have to keep begging for money, but are collectively seen as experts."
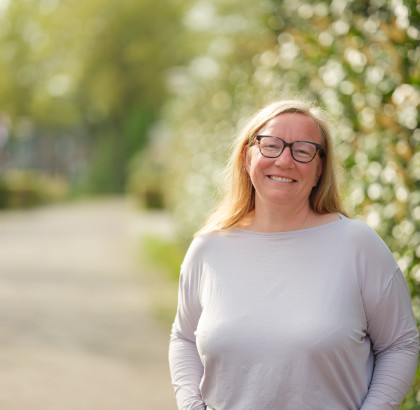
SURF agenda
-
National XR Day 2025
ConferenceErasmus Universiteit, Rotterdam, Theil building and Erasmus Building (Woudestein campus)Conference -
SURF Network & Cloud Event
ConferenceGooiland, HilversumConference -
SURF Education Days 2025
ConferenceAmare, Spuiplein 150, 2511 DG The HagueConference
Working at SURF
Working at SURF means working in an innovative organisation that plays an important role in society. Do you wish to set ambitious goals, and continue to develop your personal strengths? Then working at SURF might be just the thing for you.